Python Data Persistence – RDBMS Concepts
The previous chapter discussed various tools offered by Python for data persistence. While the built-in file object can perform basic read/write operations with a disk file, other built-in modules such as pickle and shelve enable storage and retrieval of serialized data to/from disk files. We also explored Python libraries that handle well-known data storage formats like CSV, JSON, and XML.
Drawbacks of Flat File
However, files created using the above libraries are flat. They are hardly useful when it comes to real-time, random access, and in-place updates. Also, files are largely unstructured. Although CSV files do have a field header, the comma-delimited nature of data makes it very difficult to modify the contents of a certain field in a particular row. The only alternative that remains, is to read the file in a Python object such as a dictionary, manipulate its contents, and rewrite it after truncating the file. This approach is not feasible especially for large files as it may become time-consuming and cumbersome.
Even if we keep this issue of in-place modification of files aside for a while, there is another problem of providing concurrent r/w access to multiple applications. This may be required in the client-server environment. None of the persistence libraries of Python have built-in support for the asynchronous handling of files. If required, we have to rely upon the locking features of the operating system itself.
Another problem that may arise is that of data redundancy and inconsistency. This arises primarily out of the unstructured nature of data files. The term ‘redundancy’ refers to the repetition of the same data more than one time while describing the collection of records in a file. The first row of a typical CSV file defines the column headings, often called fields and subsequent rows are records.
Following table 7.1 shows a ‘pricelistxsv’ represented in the form of a table. Popular word processors (MS Word, OpenOffice Writer) and spreadsheet programs (MS Excel, OpenOffice Calc) have this feature of converting text delimited by comma or any other character to a table.
No |
Customer Name |
Product |
Price |
Quantity |
Total |
1 |
Ravikumar |
Laptop |
25000 |
2 |
50000 |
2 |
John |
TV |
40000 |
1 |
40000 |
3 |
Divya |
Laptop |
25000 |
1 |
25000 |
4 |
Divya |
Mobile |
15000 |
3 |
45000 |
5 |
John |
Mobile |
15000 |
2 |
30000 |
6 |
Ravi Kumar |
TV |
40000 |
1 |
40000 |
As we can see, data items such as customer’s name, product’s name, and price are appearing repeatedly in the rows. This can lead to two issues: One, a manual error such as spelling or maintaining correct upper/lower case can creep up. Secondly, a change in the value of a certain data item needs to reflect at its all occurrences, failing which may lead to a discrepancy. For example, if the price of a TV goes up to 45000, the price and total columns in invoice numbers 2 and 6 should be updated. Otherwise, there will be inconsistency in the further processing of data. These problems can be overcome by using a relational database.
SQLite installation
Installation of SQLite is simple and straightforward. It doesn’t need any elaborate installation. The entire application is a self-contained executable ‘sqlite3.exe’. The official website of SQLite, (https://sqIite.org/download.html) provides pre-compiled binaries for various operating system platforms containing the command line shell bundled with other utilities. All you have to do is download a zip archive of SQLite command-line tools, unzip to a suitable location and invoke sqlite3.exe from DOS prompt by putting the name of the database you want to open.
If already existing, the SqLite3 database engine will connect to it; otherwise, a new database will be created. If the name is omitted, an in-memory transient database will open. Let us ask SQLite to open a new mydatabase.sqlite3.
E:\SQLite>sqlite3 mydatabase.sqlite3
SQLite version 3.25.1 2018-09-18 20:20:44
Enter ".help" for usage hints.
sqlite>
In the command window, a SQLite prompt appears before which any SQL query can be executed. In addition, there “dot commands” (beginning with a dot “.”) typically used to change the output format of queries or to execute certain prepackaged query statements.
An existing database can also be opened using the .open command.
E:\SQLite>sqlite3
SQLite version 3.25.1 2018-09-18 20:20:44
Enter ".help" for usage hints.
Connected to a transient in-memory database.
Use ".open FILENAME" to reopen on a persistent database.
sqlite> .open test.db
The first step is to create a table in the database. As mentioned above, we need to define its structure specifying the name of the column and its data type.
SQUte Data Types
ANSI SQL defines generic data types, which are implemented by various RDBMS products with a few variations on their own. Most of the SQL database engines (Oracle, MySQL, SQL Server, etc.) use static typing. SQLite, on the other hand, uses a more general dynamic type system. Each value stored in SQLite database (or manipulated by the database engine) has one of the following storage classes:
- NULL
- INTEGER
- REAL
- TEXT
- BLOB
A storage class is more general than a datatype. These storage classes are mapped to standard SQL data types. For example, INTEGER in SQLite has a type affinity with all integer types such as int, smallint, bigint, tinyint, etc. Similarly REAL in SQLite has a type affinity with float and double data type. Standard SQL data types such as varchar, char, char, etc. are equivalent to TEXT in SQLite.
SQL as a language consists of many declarative statements that perform various operations on databases and tables. These statements are popularly called queries. CREATE TABLE query defines table structure using the above data types.
CREATE TABLE
This statement is used to create a new table, specifying the following details:
- Name of the new table
- Names of columns (fields) in the desired table
- Type, width, and the default value of each column.
- Optional constraints on columns (PRIMARY KEY, NOT NULL, FOREIGN KEY)
Example
CREATE TABLE table_name (
column 1 datatype [width] [default] [constraint],
column2 ,
column3...,
) ;
DELETE Statement
If you need to remove one or more records from a certain table, use the DELETE statement. The general syntax of the DELETE query is as under:
Example
DELETE FROM table_name WHERE [condition];
In most circumstances, the WHERE clause should be specified unless you intend to remove all records from the table. The following statement will remove those records from the Invoices table having Quantity>5.
sqlite> select customers.name, products. name, the quantity from invoices, customers, products
...> where invoices.productID=Products.ProductID ...> and invoices.CustID=Customers.CustID;
Name Name Quantity
--------- --------- ---------
Ravikumar Laptop 2
Divya Mobile 3
Ravikumar Mobile 1
John Printer 3
Patel Laptop 4
Irfan Printer 2
Nit in Scanner 3
ALTER TABLE statement
On many occasions, you may want to make changes in a table’s structure. This can be done by the ALTER TABLE statement. It is possible to change the name of a table or a column or add a new column to the table.
The following statement adds a new column in the ‘Customers’ table:
sqlite> alter table customers add column address text (20);
sqlite> .schema customers CREATE TABLE Customers (
CustID INTEGER PRIMARY KEY AUTOINCREMENT, Name TEXT (20),
GSTIN TEXT (15), address text (20));
DROP TABLE Statement
This statement will remove the specified table from the database. If you try to drop a non-existing table, the SQLJte engine shows an error.
sqlite> drop table invoices; sqlite> drop table employees; Error: no such table: employees
When the ‘IF EXISTS’ option is used, the named table will be deleted only if exists and the statement will be ignored if it doesn’t exist.
sqlite> drop table if exists employees;
SQLiteStudio
SQLiteStudio is an open-source software from https://sqlitestudio.pl. It is portable, which means it can be directly run without having to install. It is powerful, fast, and yet very light. You can perform CRUD operations on a database using GUI as well as by writing SQL queries.
Download and unpack the zip archive of the latest version for Windows from the downloads page. Run SQLiteStudio.exe to launch the SqliteStudio. Its opening GUI appears as follows:(figure 7.3)
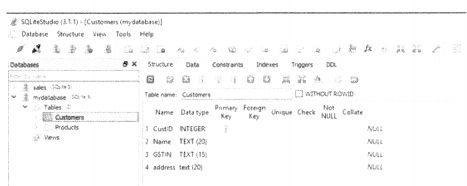
Currently attached databases appear as expandable nodes in the left column. Click any one to select and the ‘Tables’ sub-node shows tables in the selected database. On the right, there is a tabbed pane. The first active tab shows structure of the selected table and the second tab shows its data. The structure, as well as data, can be modified. Right-click on the Tables subnode on the left or use the Structure menu to add a new table. User-friendly buttons are provided in the Structure tab and data tab to insert/ modify column/row, commit, or rollback transactions.
This concludes the current chapter on RDBMS concepts with a focus on the SQLite database. As mentioned in the beginning, this is not a complete tutorial on SQLite but a quick hands-on experience of interacting with SQLite database to understand Python’s interaction with databases with DB-API that is the subject of the next chapter.